Review of feature selection approaches based on grouping of
5 (463) In stock
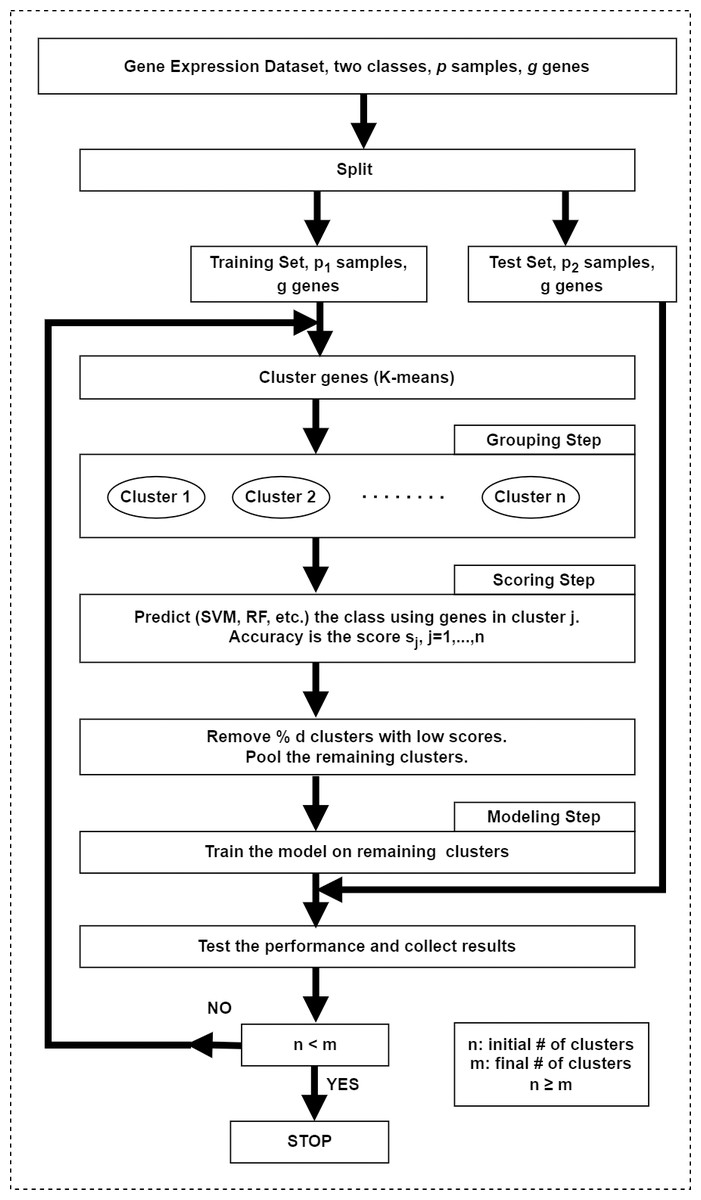
With the rapid development in technology, large amounts of high-dimensional data have been generated. This high dimensionality including redundancy and irrelevancy poses a great challenge in data analysis and decision making. Feature selection (FS) is an effective way to reduce dimensionality by eliminating redundant and irrelevant data. Most traditional FS approaches score and rank each feature individually; and then perform FS either by eliminating lower ranked features or by retaining highly-ranked features. In this review, we discuss an emerging approach to FS that is based on initially grouping features, then scoring groups of features rather than scoring individual features. Despite the presence of reviews on clustering and FS algorithms, to the best of our knowledge, this is the first review focusing on FS techniques based on grouping. The typical idea behind FS through grouping is to generate groups of similar features with dissimilarity between groups, then select representative features from each cluster. Approaches under supervised, unsupervised, semi supervised and integrative frameworks are explored. The comparison of experimental results indicates the effectiveness of sequential, optimization-based (i.e., fuzzy or evolutionary), hybrid and multi-method approaches. When it comes to biological data, the involvement of external biological sources can improve analysis results. We hope this work’s findings can guide effective design of new FS approaches using feature grouping.
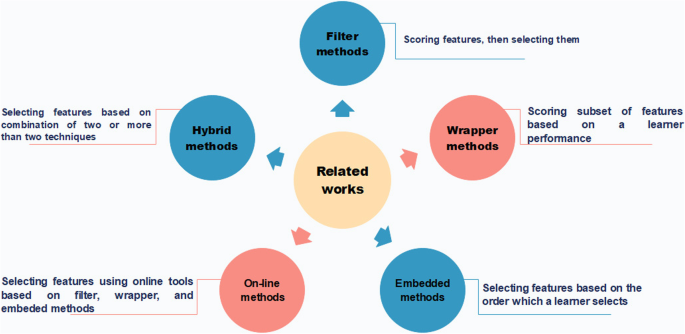
FeatureSelect: a software for feature selection based on machine

The architecture of the framework. Each processing step is encapsulated
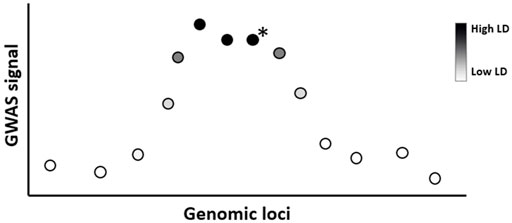
Frontiers A Review of Feature Selection Methods for Machine
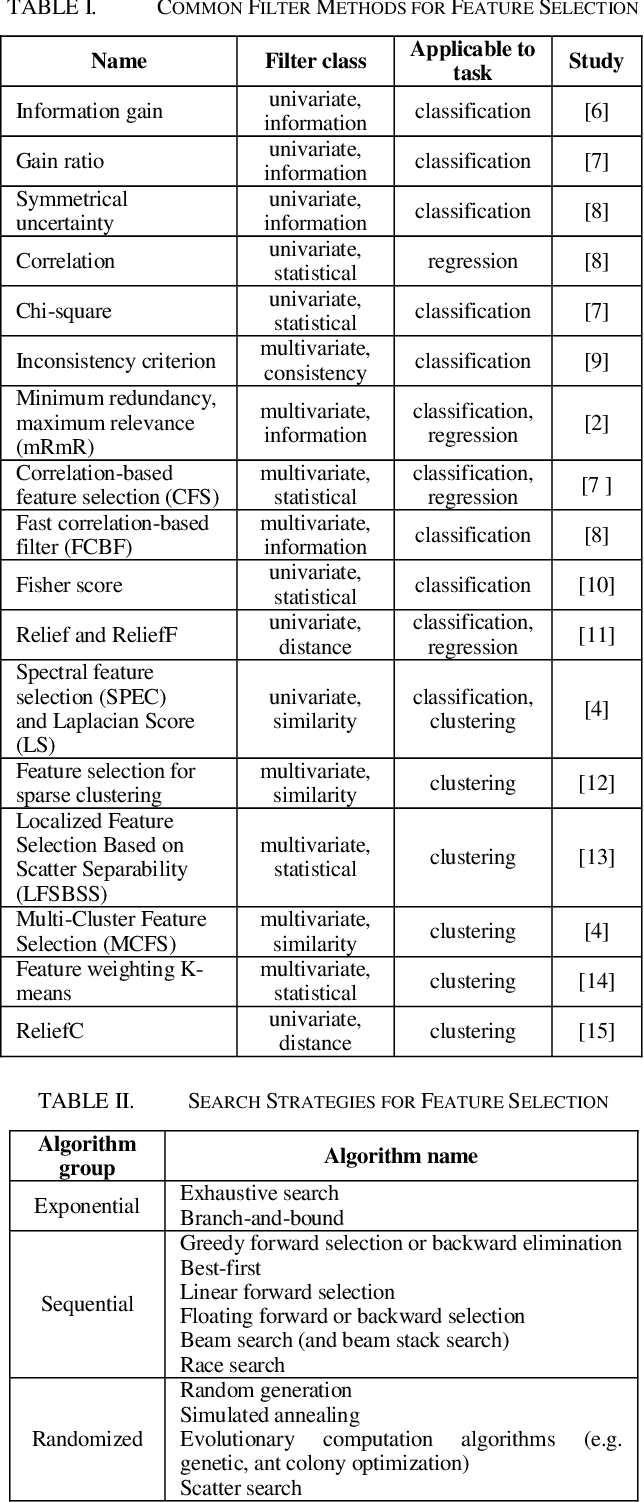
PDF] A review of feature selection methods with applications
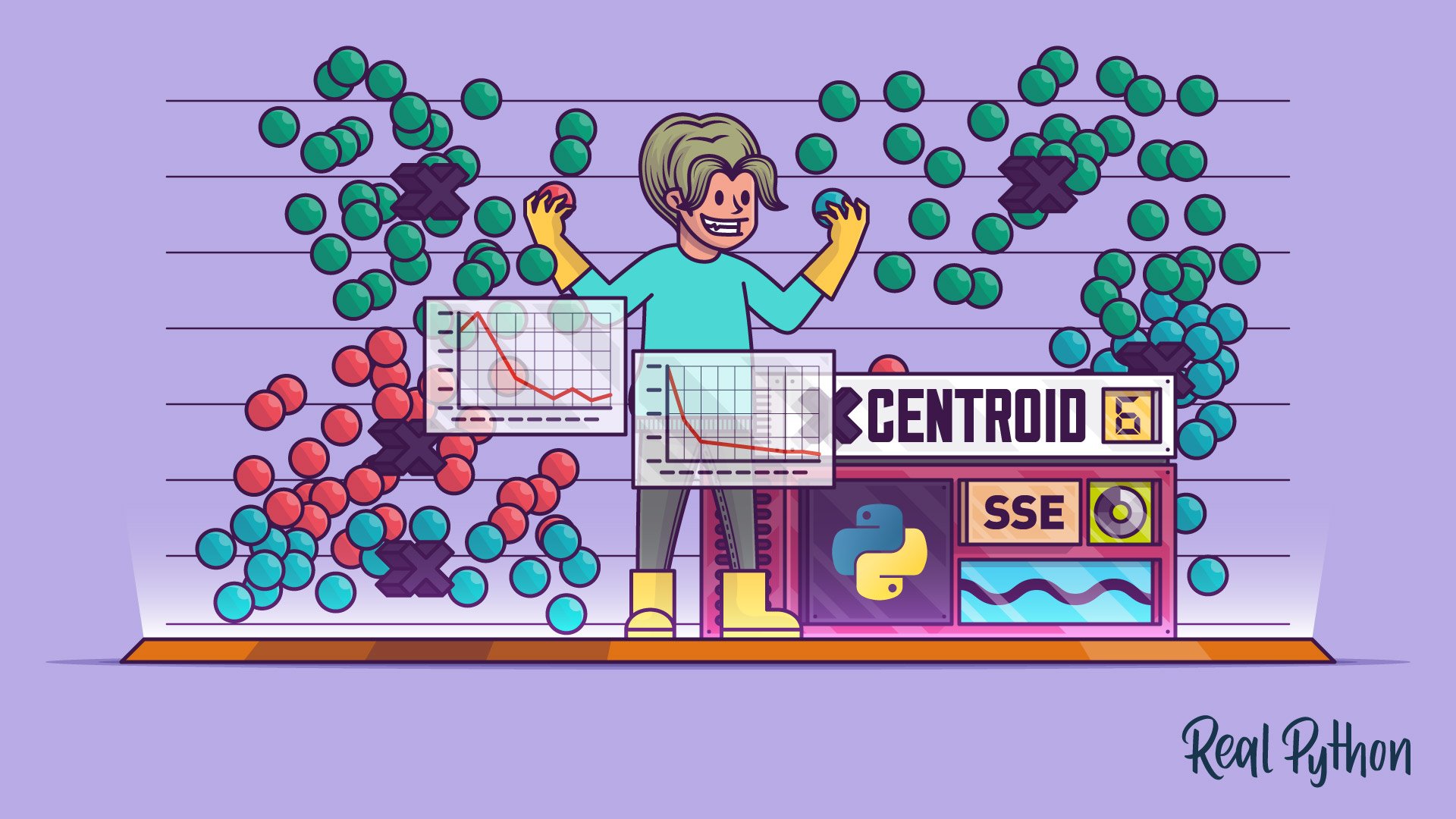
K-Means Clustering in Python: A Practical Guide – Real Python

GediNET for discovering gene associations across diseases using knowledge based machine learning approach. - Abstract - Europe PMC
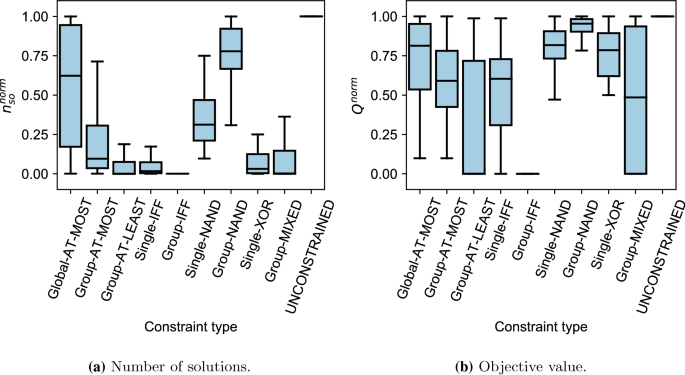
An Empirical Evaluation of Constrained Feature Selection

Mean subset size for the eight feature selection methods.
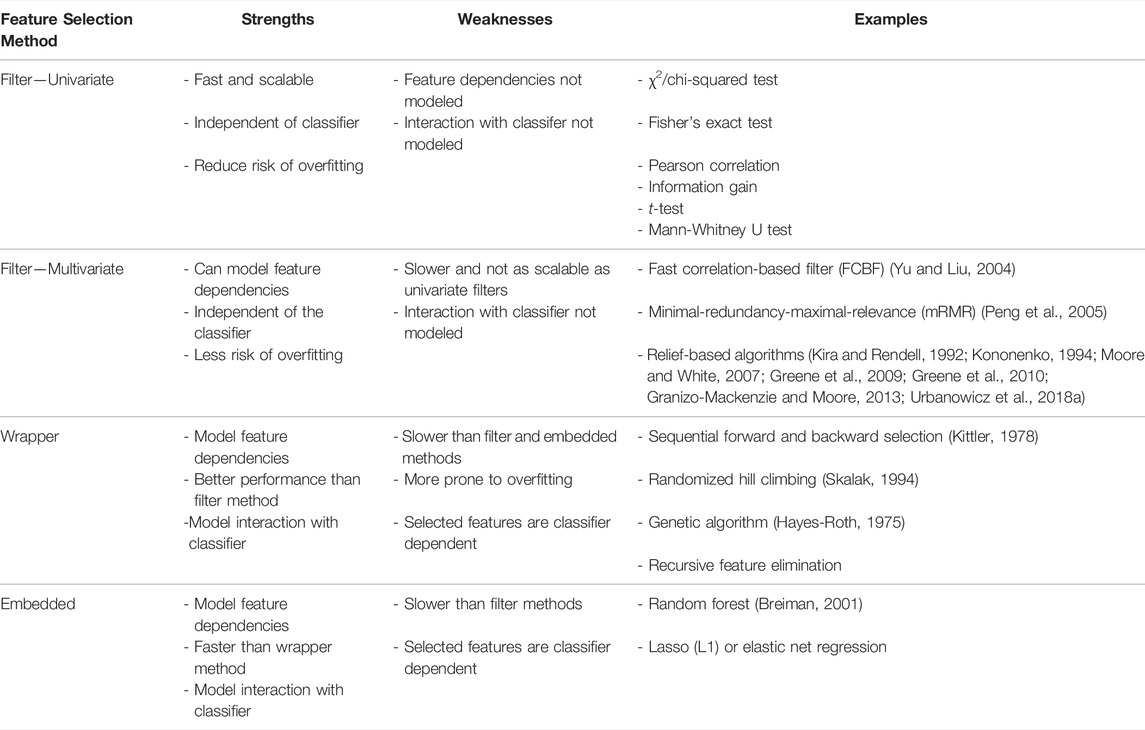
Frontiers A Review of Feature Selection Methods for Machine

Overview on traditional gene selection approaches and their
www.weisfeedback.com Weis Feedback Survey
1. Issues in the Measurement of Criminal Careers, Criminal Careers and Career Criminals,: Volume II
- Golf Clubs, Drivers, Irons, Woods, Hybrids, Wedges, Putters & Packages
- Stretch Faux Leather Fabric
- Be Thankful And Happy Thanksgiving Day Digital Art by Anh Phan - Pixels Merch
- Lil'Posh Resale Kid's Boutique on Instagram: Just in! New! Bellefit Dual Closure Girdle in XL, medical device that allows Recovery & Treatment adter c section, natural birth, Medical Grade, retail: $145, our
- Calcinha Puket Caleçon Algodão Feminina Unicórnio Love
- SPANX Suit Your Fancy Plunge Low-Back Mid-Thigh Bodysuit